Demystifying Demand Forecasting
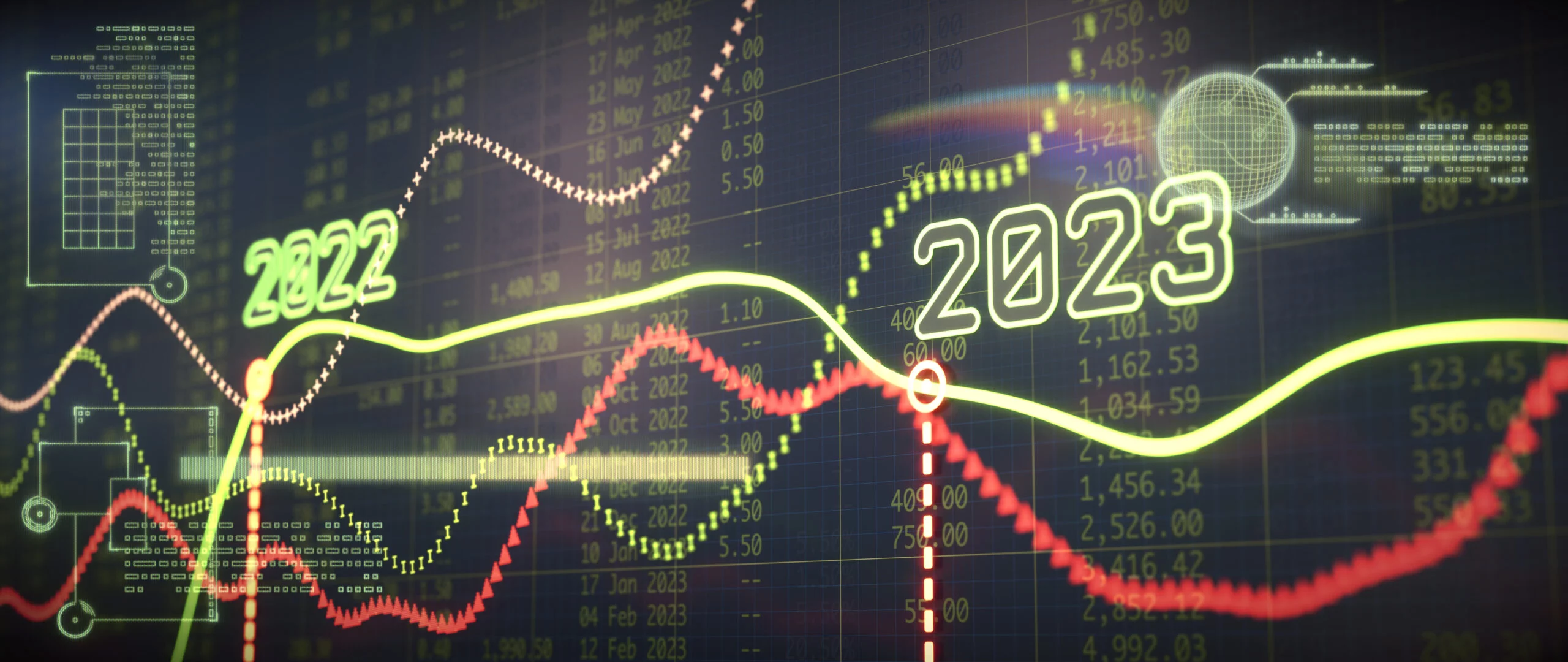
Right now, the distributed energy resource (DER) and electric vehicle (EV) markets are experiencing exponential growth, whether as utilities work to meet decarbonization goals, due to increased EV manufacturing, or rising public interest in renewables. These new grid technologies create additional demand, though they likewise add potential grid assets for enterprising utilities. As such, quickly and efficiently determining how much electric demand an area might need on a given day is a complicated, but surmountable challenge. Fortunately, demand forecasting technology is designed to predict and interpret potential demand, allowing utility program managers to plan accordingly in advance.
A Practical Look At Demand Forecasting
Any demand forecasting method considers several factors, from historical demand to the continuously evolving influence of the weather. Demand forecasting offers utilities the opportunity to parse these variables to their advantage. Suppose a program manager wants to dig a little deeper and look at how the demand might play out on a smaller scale. The right load forecasting technology will help illustrate how will energy usage changes throughout the day as temperatures rise and fall.
For example, suppose the weather forecast predicts tomorrow will be cold and rainy. In that case, your load forecasting model may account for increased power usage as people heat their homes and businesses. Likewise, that data confirms for program managers that the use of renewable DER assets via virtual power plants or other demand flexibility programs isn’t viable to strengthen grid resilience if needed, leaving any demand needs left to energy markets.
Why Demand Forecasting Is Important
Demand forecasting helps energy companies determine how much electricity they should generate or buy to meet consumer demand. Accurate load forecasting does much more than “keep the lights on,” though. Reliable forecasting helps:
- Reduce fossil fuel emissions by minimizing overall usage either by relying on renewable energy assets or by utilitizing the most affordable TOU rates possible.
- Keep costs affordable by minimizing unnecessary energy expenditures, while stabilizing rates.
- Plan for the evolving needs of the areas they serve.
- Maintain infrastructure assets to avoid outages and expensive replacements.
Accurate demand forecasting benefits everyone, including companies that want to minimize costs and consumers who want reliable access to electricity.
Demand Forecasting Time Horizon Classes
Energy providers want to know how much power an area needs today, but they also need to know what to expect years from now. To do that, artificial neural networks and similar technologies are employed to measure different lengths of time to generate the most up-to-date and accurate predictions possible. Demand forecasting has four classes based on their time horizons:
- Very Short Term Forecasting (VSTF) looks at demand within the next few milliseconds to the next few hours, primarily to determine how much renewable energy sources will contribute to the grid.
- Short Term Forecasting (STF) predicts demand over the next few days so companies know how much energy they need to meet the area’s demand.
- Medium Term Forecasting (MTF) looks at the next few days and months to help companies maintain grid resilience.
- Long Term Forecasting (LTF) can predict trends years in advance to help grid managers prepare for growth
Over the last two decades, researchers have increasingly focused on STF and LTF, likely because they have such practical implications for energy providers.
Popular Approaches to Demand Forecasting
It should go without saying, but there are a variety of ways to approach demand forecasting. This includes countless factors that can affect load forecasting, including artificial intelligence (AI), machine learning (ML), and deep learning (DL).
Deep Neural Networks (DNNs)
Deep neural networks take a multilayered approach to forecasting energy consumption. It makes sense to use DNNs because they can incorporate historical data into their predictions. They can also learn from their mistakes, which helps minimize inaccuracies over time.
There are also downsides to using DNNs. They need access to massive amounts of data, which means they can take a long time to produce accurate outcomes. Processing that much data also requires enormous resources, including graphical processing units (GPUs) and electricity to power them.
Convolutional Neural Networks (CNNs)
Convolutional neural networks suit load forecasting because they can include millions of weighted values. CNN also benefits from including the results of mathematical operations in future calculations. This is necessary when working with an organic system that includes weather patterns and human behaviors because you uncover how variables influence each other over time.
Since convolution can seem incredibly abstract to the human brain, you can think of it in simpler terms by imagining some basic math rules. For example, when solving the equation X = (1-Y) + (2-Y), you would complete the parts inside the parentheses and replace them with results. Similarly, CNN for load forecasting includes nested variables that feedback to the larger algorithm.
Recurrent Neural Networks (RNNs)
Recurrent neural networks rely on connected nodes that use internal memory to communicate with each other. A node located later in the algorithm can send its results to a node located earlier in the algorithm. The network, therefore, can feed back data circularly and adjust to changing complex conditions.
The Future of Demand Forecasting
Training neural networks would present one path forward with demand forecasting, especially by employing fuzzy logic, which could make it possible for algorithms to teach themselves without as much human oversight. Fuzzy logic uses a collection of loose rules with varying levels of accuracy. As the algorithm makes and tests predictions, it can reform those rules to create more accurate forecasts in the future. In some cases, like Peru, already use fuzzy systems for short-term demand forecasting. It produces better results than conventional forecasting methods, and it has the potential to improve the accuracy of medium-term and long-term forecasting.
Researchers still have plenty of work ahead of them before they build a system that independently predicts electricity needs flawlessly. Considering how much progress has been made over the last couple of decades, though, the future looks promising. As the technology improves, it should give energy providers better insight into how they can curb fossil fuel emissions, lower costs, and maintain grids.
Demand Forecasting Conclusion
The opportunities that demand forecasting presents for utility program managers are vast. By preparing for tomorrow, program managers can better utilize their existing assets, whether that entails finding the lowest possible energy to purchase on the market, or engaging your customers to prepare them for the potential of a demand flexibility event like demand response or managed EV charging, or in running a DER program like a virtual power plant or battery program. In doing so, program managers can reduce reliance on expensive energy costs AND meet decarbonization goals at the same time.